Increasing Trust in Rating and Review Systems Through Data
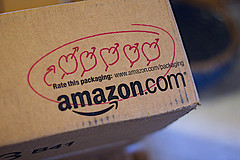
Credit: Alan Levine (CC BY 2.0)
With Fandango’s sabotaging the credibility of their own user ratings and Amazon suing more than 1,000 Fiverr users for posting fake product reviews, I thought it would be fun to talk about building trust in review systems.
If people can’t trust your reviews, you may as well not have them at all.
Having a code of conduct you actually enforce is important. But let’s think about this in a bigger sense, focusing on 3 key ideas that have to do with the systems you use and the data they have access to.
Verifying Purchase/Use
Reviews are more valuable when you know that the person reviewing the product has actually used it – or, at least, purchased it. Amazon does this, where reviews are tagged “verified purchase.” Those reviews enjoy enhanced credibility, and I can see a day when Amazon allows you to view the star rating given by verified purchasers only.
Of course, purchase doesn’t mean use. There are people gaming the system by giving people Amazon coupons to “purchase” the item for free, in exchange for a review. Then it is a “verified purchase,” even if it might not be the most credible review. As long as the freebie is disclosed, it’s not a big deal. But, on the whole, a verified purchase does make it more likely that the review is coming from someone who has actually experienced the product. Which is very good.
What if you could verify use, to a reasonable extent? Let’s stick with Amazon and consider their Kindle reading devices. What if they showed you book reviews by people who they knew had the book open for more than an hour? Or that they knew read at least X number of pages and spent at least X number of minutes per page? Yes, everything can be gamed. They could just leave their reading device on and not read it. But not many would do that. Reviews from people that you know spent time with the book are more valuable.
Look at Netflix. They know how long you watched a movie – or they know how long the movie played, anyway. What if they not only showed ratings of the movie, but ratings and reviews of the movie by the accounts they know at least played more than half of it?
Uber ratings are interesting because you know they used the service. The driver had that rider and the rider experienced that driver. You can apply this to all sorts of apps that are an intermediary between two parties. Restaurants, hotels, etc. A review from someone you know stayed (or, at least, was billed for the stay) at the hotel is more valuable than a review from some random person.
Of course, not many will have access to this data. But if you do, it’s a tremendous asset.
Weighted Averages
IMDb and Metacritic do this. The idea is that some reviewers are more credible than others. You just have to develop a formula for calculating this. A math expert or two might come in handy here.
But to think about it loosely, a long term member of your community who regularly rates movies, and whose reviews have received many helpful votes, should have a bigger voice than someone who just registered to rate one movie. That person is more likely to be rating simply to skew something higher or lower, not to actually provide a balanced opinion of the film. The new reviewer can build up credibility, just like the veteran member has.
Forensic Science
We collect information from our members that can be identifying, such as IP addresses, email addresses, website links and more. But we don’t really use this information proactively. If we notice a trend or have one reported to us, we might look into it. We might even be able to search for accounts and contributions by IP address. But we don’t really spend much time with that data.
I would build a system where your software automatically identifies trends for you and flags them. While hand checking IPs on every contribution is out of the question, if the system notices that the same IP reviewed the same movie twice, that’s something you might want to be notified about. Just like if surfercoolguy111@gmail.com and surfercoolguy111@yahoo.com reviewed it. They don’t have the same email address, but the likelihood of two different people, using a statistically improbable handle in their email address and then reviewing the same movie on your site, is fairly slim.
This data is very tedious to sort through, which is why you want an automated system flagging it, so that you can spend your time taking action.
If you host reviews and ratings, what are you doing to make them more credible? Please let me know in the comments.